
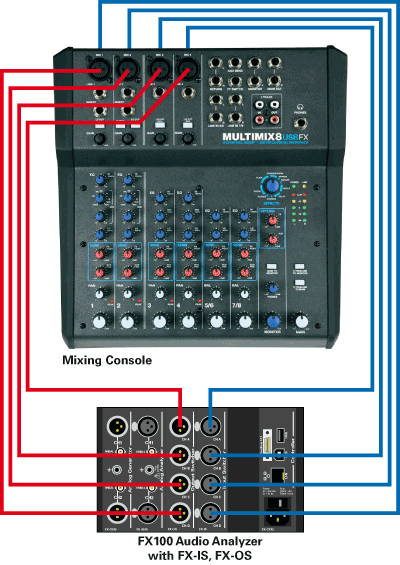
In both of these common scenarios there are strong pressures to stop the test early and call it either way. These fixed-sample tests, as they are called, are inefficient and impractical as using them means sticking to a predetermined number of exposed users regardless if the performance of the tested variant looks disastrous or super good when examined in the interim.
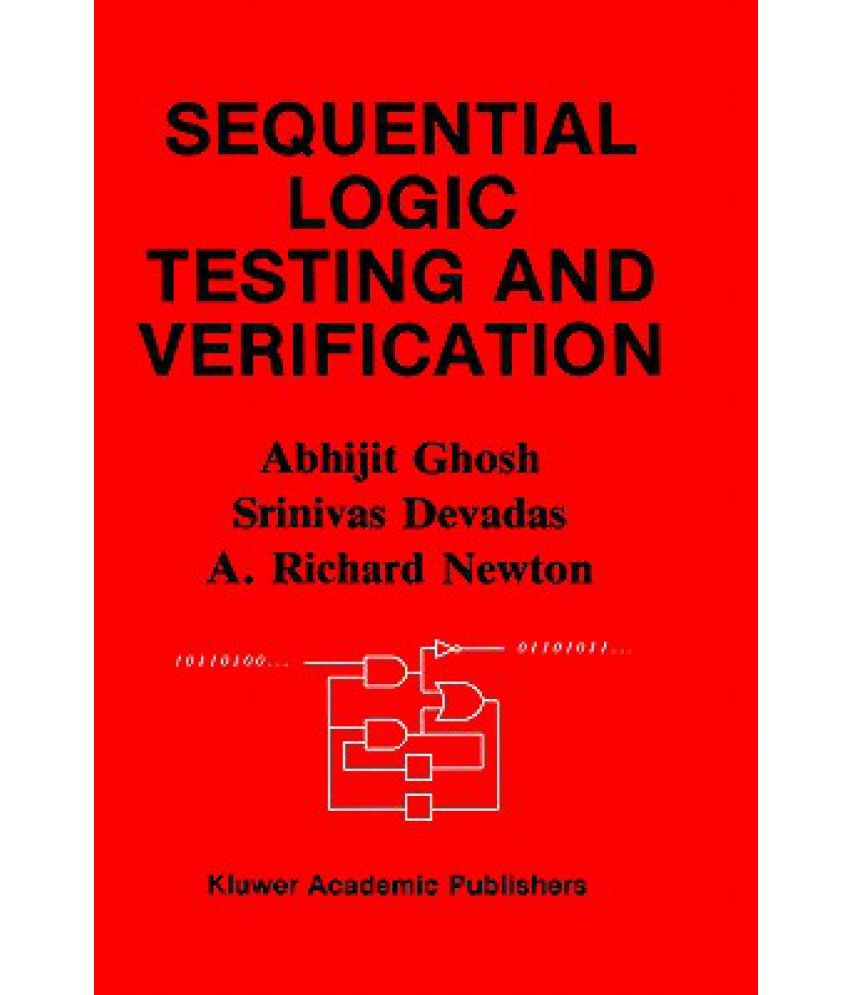
How this works is that one determines the test’s sample size or duration up front, then once that sample size or duration is reached a p-value and confidence interval are computed and a decision is made if they meet the desired error level. Classic statistical tests control these error levels under the key assumption that the data is analyzed just once. In frequentist tests this is done through controlling the false positive rate and the false negative rate. In any statistical test, we decide on a level of error we are willing to accept. Lucia: Can you explain how sequential statistics work ‘for dummies’? One tool after another, over time the platform shifted towards offering a suite of statistical tools for planning and analysis of A/B tests distinguished by the statistical rigor and the transparency in the methods employed, as well as being designed in a way that minimizes the possibility of misuse and misinterpretation of statistical methods.
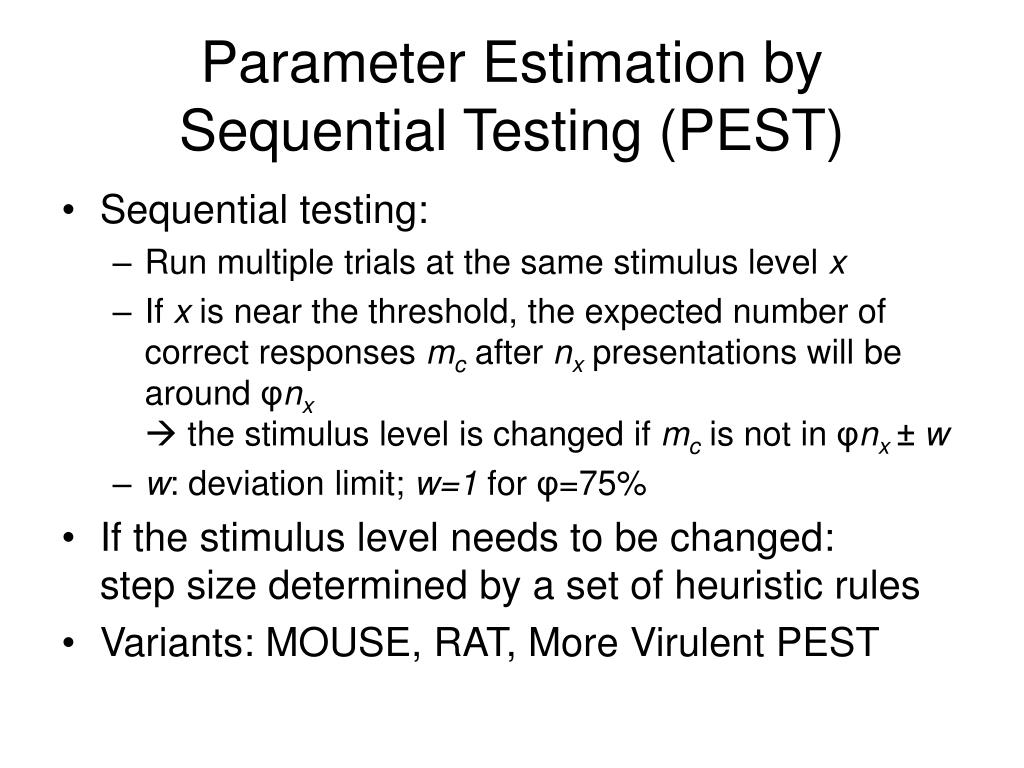
“waiting for significance”) and how that inflates the false positive rate, I began work on what is now known as AGILE and shortly after introduced my first implementation of frequentist sequential testing tailored to CROs. Likewise, when I started talking about the issue of peeking on test outcomes with intent to stop a test (a.k.a. This allowed users to perform analyses of A/B/N tests with rigorous control of the false positive rate at a time where pretty much no other tool offered it. testing B v A, C v A, and D v A, separately), I added a statistical significance calculator with multiple comparisons adjustments. For example, after discussing the issue of multiple comparisons where people were incorrectly analyzing A/B/N tests by separate pairwise comparisons (e.g. I wasn’t satisfied with just that as I wanted to also offer solutions to the problems that I covered. As I was noticing significant issues with how statistics were applied in the A/B testing practice, I started to point them out. My focus, however, soon shifted from web analytics to statistics and in particular – statistical analyses of online A/B tests. Georgi: Initially it was a project aimed at helping Google Analytics professionals automate GA-related tasks at scale, as well as enhance GA data in various ways. In my answers I draw on my experience as an early advocate for the adoption of sequential statistics, as a developer implementing these methods at Analytics Toolkit, as well as an advisor on the introduction of sequential testing at ABSmartly’s A/B testing platform. In this short interview she asks questions from the view of a CRO practitioner and their clients and stakeholders. For this article I teamed up with Lucia van den Brink, a distinguished CRO consultant who recently started using Analytics Toolkit and integrated frequentist sequential testing into her client workflow. Sequential statistics are gathering interest and there are more and more questions posed by CROs looking into the matter.
